AI Search Behaviour Analysis Agent: A Comprehensive Guide to Behaviour Analysis and Analytics
Artificial intelligence (AI) is fundamentally transforming how organisations interpret user behaviour analysis, empowering more informed decision-making and enhancing customer experience.
This guide presents an in‐depth exploration of the AI agent concept known as a search behaviour analysis agent. We examine advanced behavior analysis AI methodologies, evaluate cutting-edge analytics tools, and review practical use cases that enable businesses to leverage machine learning for superior customer behaviour analysis.
Crafted in the distinctive Geeky Tech style, this article is an educational resource designed to enrich both technical understanding and strategic application.
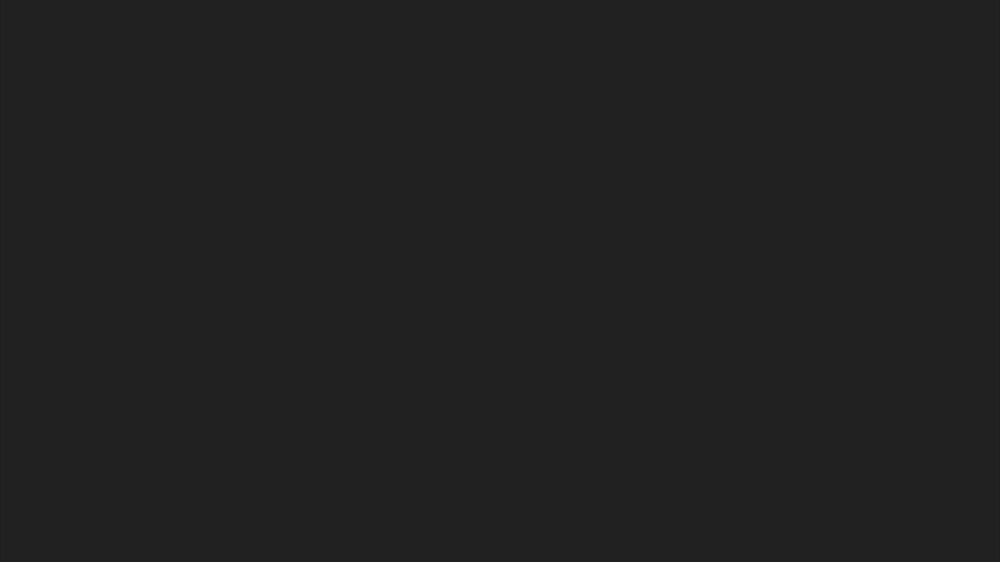
How Our Clients Did Last Month
Month-over-month traffic trends. Source: Google Search Console
AI Agents For Customer Behavior Analysis on Search Engines
AI technology is redefining digital landscapes through sophisticated data analysis that delivers actionable insights into user interactions. This guide critically examines the AI search behaviour analysis agent from technical and strategic perspectives, outlining its role in optimising digital strategies and personalising user experiences.
Key topics include:
- The definition and evolution of AI search behaviour analysis
- Detailed examinations of machine learning methodologies and predictive analytics that underpin user behaviour analysis
- Real-world use cases demonstrating measurable enhancements in customer engagement and customer satisfaction
Understanding the Basics of User Behavior Analysis with AI
What is an AI Search Behaviour Analysis Agent?
An AI search behaviour analysis agent is a specialised AI system that employs advanced analytics and data analysis to track and analyze customer behaviour.
By monitoring user interactions across digital platforms, these agents generate deep insights into how users interact with websites and applications. Through the combined use of machine learning, natural language processing, and predictive analytics, the agent delivers real-time data that supports both optimisation and personalisation strategies.
Evolution from Traditional Analytics
Conventional log analysis and manual analytics methods have given way to systems offering in-depth analysis via sophisticated AI. Contemporary AI search behaviour analysis agents harness extensive data sets and cutting-edge AI to convert raw data into strategic business intelligence.
These systems deploy advanced analytics solutions that transform complex information into actionable insights, enabling organisations to analyze customer behaviour with remarkable precision.
Key Terminology
- Artificial Intelligence (ai) and AI models
- Behaviour analysis vs. behavioral analysis (both spellings are employed in this guide)
- Customer behaviour analysis and user behaviour analysis
- Predictive analytics, real-time analytics, and log analysis
- Machine learning, deep learning, and neural network (machine learning)
- Data analysis and web analytics
The Mechanics of AI Search Behaviour Analysis Agents
Detailed Technical Overview
An AI search behaviour analysis agent integrates several advanced technical components. The process involves multiple stages that convert raw data into strategic insights:
Data Collection & Integration
- Real-Time Data: The agent aggregates real-time data from diverse digital touchpoints utilising techniques similar to google analytics and other customer behaviour analytics tools.
- Multi-Source Integration: It consolidates information from web interactions, mobile app usage, and offline customer engagements to ensure comprehensive data collection.
Algorithm Deployment & Model Training
- Machine Learning Framework: Ai models are deployed and continuously refined through supervised learning, reinforcement learning, and selective application of deep learning techniques. Comprehensive training, validation, and test data sets ensure high accuracy and precision.
- Predictive Analytics: Advanced algorithms identify trends and anomalies in user behaviour analysis, utilising predictive analytics to forecast future customer interactions and consumer behaviour.
Data Processing & Analysis
- In-Depth Analysis: The agent performs a comprehensive analysis using robust analytics solutions that convert raw figures into actionable insights. Techniques such as log analysis and advanced data visualisation are central to understanding the customer journey.
- Real-Time Alerts: The system triggers immediate alerts based on key performance indicators and identified bottlenecks, enabling prompt decision-making.
Reporting & Visualisation
- Infographics and Dashboards: Visual tools succinctly depict the transformation from data collection to actionable insights, clarifying how user behaviour analysis drives strategic outcomes.
Implementation Best Practices
To ensure the successful deployment of an AI search behaviour analysis agent, consider these best practices:
Integration with Existing Systems:
Outline clear steps for integrating AI technology with current web analytics and customer behaviour analysis tools. Use strategies that streamline data collection and facilitate smooth interoperability.Incremental Deployment:
Implement the system gradually, starting with non-critical applications. This phased approach allows organisations to validate the system’s accuracy and refine predictive analytics models using real-world data.Staff Training and Change Management:
Invest in comprehensive training for teams responsible for managing and interpreting analytics. This ensures both technical and non-technical staff are equipped to use the AI system effectively for analyzing customer behaviour and making informed decisions.Ongoing Monitoring and Maintenance:
Regularly audit AI models to ensure continued accuracy and compliance with regulatory standards. Emphasise the importance of explainable artificial intelligence to maintain transparency and build trust.
Pitfalls and Challenges to Avoid
While the benefits are substantial, avoiding common pitfalls is essential:
Overreliance on Automation:
Even with advanced real-time analytics and automated log analysis, human oversight remains crucial to catch subtle anomalies that algorithms might miss. A balanced approach that combines automation with expert review ensures optimal performance.Insufficient Training Data:
Poor-quality or limited training data can result in suboptimal AI models. Organisations must invest in robust, diverse data sets to enhance predictive analytics capabilities and reduce the risk of bias.Neglecting Privacy and Compliance:
Failure to adhere to regulatory compliance such as GDPR can lead to significant legal and reputational challenges. Always prioritise data privacy, transparency (behaviour), and rigorous audit processes.Ignoring Scalability:
Implementing solutions that cannot scale effectively may lead to bottlenecks. It is important to choose analytics solutions that grow with your customer base and evolving data volume.
Comparative Analysis of Tools
A comprehensive understanding of available solutions is vital. When comparing various user behaviour analytics software options, consider these factors:
Data Integration Capabilities:
Evaluate how well the tools consolidate data from multiple sources, including web, mobile, and offline interactions, to provide a unified data analysis framework.Accuracy and Real-Time Processing:
Assess the speed and precision of real-time analytics and predictive analytics capabilities, ensuring that the solution can deliver timely actionable insights.User Interface and Visualisation:
A user-friendly interface with clear data and information visualisation dashboards enhances the ability to understand and act on user behaviour analysis.Scalability and Customisation:
Ensure the solution can be tailored to meet specific business requirements and can scale seamlessly as data volumes increase.Cost and Support:
Consider the pricing model and level of customer support available. A free trial option is often beneficial to assess the tool’s fit for your needs before full implementation.
Metrics and KPIs for Success
To measure the success of your AI search behaviour analysis agent, focus on key performance indicators such as:
Conversion Rates:
Monitor changes in conversion metrics post-implementation to gauge improvements in customer engagement and the effectiveness of personalised content strategies.Customer Satisfaction and Loyalty:
Evaluate feedback from customer support channels, repeat visit rates, and overall customer satisfaction to understand the impact on customer behaviour analysis.Operational Efficiency:
Track the reduction of bottlenecks and improvements in data analysis turnaround times as a measure of increased operational efficiency.Accuracy of Predictive Analytics:
Assess how effectively the AI models forecast user interactions and detect anomalies through real-time alerts. This KPI can be crucial for optimising decision-making and maintaining high-quality analytics.
Real-World Applications & Use Cases
Industry Challenges and Geeky Tech Solutions
Organizations across various sectors face similar challenges that can be effectively addressed through AI search behaviour analysis agents:
Retail Sector Challenges:
Retailers often struggle with high rates of abandoned shopping carts and inconsistent customer engagement. Geeky Tech employs predictive analytics and comprehensive customer behaviour analysis tools to diagnose these issues. By leveraging real-time data and advanced machine learning techniques, Geeky Tech helps optimise the customer journey, ultimately boosting conversion rates and customer loyalty.Financial Services Challenges:
In financial services, institutions must balance stringent security requirements with the need for seamless user behaviour analysis. Common challenges include fraud detection and maintaining computer security while ensuring efficient customer support. Geeky Tech utilises detailed log analysis and predictive analytics to detect anomalies early and streamline operations without compromising on data privacy or regulatory compliance.E-Commerce Challenges:
E-commerce platforms frequently encounter difficulties in delivering personalised experiences at scale, resulting in lower user engagement and sales conversion rates. Geeky Tech addresses these issues by integrating AI search behaviour analysis agents with robust web analytics systems. This integration provides granular insights into customer behaviour and preferences, allowing businesses to tailor product recommendations and improve overall user experience.
Tools and Technologies
Web Analytics Software:
Tools such as google analytics and specialised user behaviour analytics software integrate with AI technology to offer comprehensive data analysis.Behavioural Analytics Tools:
These advanced tools perform meticulous in-depth analysis of customer data and user interactions, generating actionable insights that underpin effective optimisation.
Future Trends in AI & Search Behaviour Analysis
Emerging Technologies
The field of AI search behaviour analysis is in constant evolution. Advancements in ai, including further refinements in deep learning and reinforcement learning, are poised to enhance predictive analytics even further. Emerging trends include:
- Greater automation in data analysis processes
- Expanded utilisation of natural language processing to decode complex user interactions
- Broader deployment of cloud computing solutions to support more robust real-time analytics
Predicted Shifts in Analytics
As AI technology matures, the realms of business intelligence and marketing strategy will increasingly rely on real-time analytics to elucidate customer behaviour and preferences. This evolution will empower organisations to better personalise digital strategies and elevate both customer engagement and operational efficiency.
Strategic Implications
- Optimisation of Data Pipelines:
Organisations should refine their data collection and analytics solutions to eliminate processing bottlenecks and boost operational efficiency. - Investment in Advanced Analytics:
Emphasis should be placed on deploying robust AI models and predictive analytics to maintain a competitive edge in user behaviour analysis. - Focus on Ethical AI:
A sustained commitment to monitoring and auditing AI systems is essential to comply with regulatory standards and address concerns related to bias and transparency (behaviour).
Unique Perspectives and Strategic Insights
Ethics and Transparency in AI Behaviour Analysis
Organizations must deploy AI technology responsibly by ensuring that ethical frameworks are in place to mitigate bias and promote explainable artificial intelligence.
This section explores strategies for maintaining transparency (behaviour) in data analysis, detailing methods to communicate clearly how analytics insights are generated. Regulatory compliance with standards like the General Data Protection Regulation (GDPR) is also a key focus, ensuring that customer data is handled with utmost integrity.
Integration with Emerging Technologies
Modern solutions often integrate AI search behaviour analysis agents with other innovative tools such as voice search, chatbots, and augmented reality.
By merging insights from user behaviour analysis across these technologies, organizations can gain a more holistic view of customer behaviour. This section examines how Geeky Tech leverages such integrations to offer comprehensive analytics solutions, enabling businesses to personalise experiences in novel ways.
Cross-Channel User Engagement
A unique challenge for many businesses is the need to unify insights from multiple digital channels. This section discusses how multi-channel analytics can consolidate data from web, mobile, social media, and even offline interactions to provide a unified view of the customer journey.
With unified dashboards and holistic reporting, Geeky Tech helps organisations tailor their strategies to enhance both customer engagement and user experience.
Behavioural Economics and AI
Incorporating principles from behavioural sciences and behavioural economics, this section explores how AI not only predicts but also influences consumer behaviour.
Insights derived from user behaviour analysis can inform marketing strategies that drive customer loyalty and influence decision-making. Geeky Tech applies these insights to create incentive structures and personalised experiences that resonate with individual customer needs.
Real-World Integration Challenges and Success Stories
Rather than focusing on specific company names, this section addresses the common challenges that organizations face when transitioning from traditional methods to AI-driven analytics.
Typical issues include fragmented data analysis, integration difficulties with legacy systems, and ensuring data quality for effective machine learning.
Geeky Tech’s approach involves meticulous log analysis, continuous monitoring, and customised analytics solutions that overcome these obstacles, ultimately improving customer engagement and operational efficiency.
Future-Ready Strategies
This forward-looking section provides expert forecasts on the evolution of AI models and predictive analytics. It outlines innovative approaches to staying ahead in user behaviour analysis, including investment in emerging technologies and continuous system optimisation.
Recommendations are provided for preparing for future disruptions, ensuring that businesses remain agile and responsive to rapid changes in the analytics landscape.
Frequently Asked Questions
What is an AI search behaviour analysis agent?
An AI search behaviour analysis agent is an advanced AI system that employs analytics and machine learning to analyze customer behaviour.
It systematically tracks and interprets user interactions across various platforms to generate actionable insights that drive strategic decisions.
The system integrates predictive analytics with real-time data processing, enabling businesses to adapt quickly to evolving customer needs. This comprehensive approach not only supports personalisation but also enhances overall customer experience.
How does AI improve search analysis?
By leveraging advanced AI models and predictive analytics, an AI search behaviour analysis agent processes real-time data from diverse digital sources to provide a comprehensive view of user behaviour.
This method facilitates detailed log analysis and transforms raw data into meaningful insights, which can be used to tailor content and optimise digital strategies.
The system continuously refines its predictions by learning from machine learning algorithms, ensuring that businesses can respond dynamically to emerging trends. This results in more effective and responsive digital strategies that improve customer engagement.
What role does machine learning play in behaviour analysis?
Machine learning is a critical component of an AI search behaviour analysis agent, enabling the system to process extensive data and detect subtle patterns in user behaviour analysis.
It forms the backbone of predictive analytics, allowing the agent to forecast future customer interactions and identify potential issues before they become bottlenecks. By continuously learning from new training data, the system improves its accuracy and ensures that data analysis remains robust.
This iterative process helps businesses make more informed decisions and better understand the intricacies of customer behaviour.
How can businesses mitigate challenges in deploying AI for behavioural analysis?
Businesses can overcome deployment challenges by investing in high-quality training data and ensuring that their AI models are both accurate and explainable.
Regular audits and transparent reporting mechanisms are essential to maintain regulatory compliance and address concerns around data privacy and bias. Additionally, a balanced approach that combines real-time analytics with human oversight can help detect and rectify anomalies that automated systems might miss.
By integrating these strategies, organisations can successfully implement AI-driven analytics to enhance their customer behaviour analysis.
What are the benefits of a free trial in user behaviour analytics software?
A free trial offers businesses the opportunity to evaluate the performance and suitability of user behaviour analytics software before making a full commitment.
It allows organisations to experience firsthand the benefits of real-time data processing, detailed log analysis, and the generation of actionable insights. During the trial, companies can assess how effectively the solution personalises user experiences and enhances customer engagement.
This trial period is a valuable way to determine whether the software meets specific operational needs and can be scaled to address future challenges.
How does deep learning enhance behavioural analysis?
Deep learning techniques enable an AI search behaviour analysis agent to process highly complex data patterns, significantly improving the accuracy of predictive analytics. By breaking down intricate data sets, deep learning allows for a deeper analysis of user interactions and more precise data analysis.
This advanced capability ensures that subtle trends in customer behaviour are not overlooked, leading to more robust and reliable insights. The outcome is a more finely tuned system that enhances personalisation and supports proactive, informed decision-making.
Glossary of Key Terms
- Artificial Intelligence (ai): Technology that simulates human intelligence processes using computer systems.
- Ai Agent: A specialised system designed to perform tasks using AI technology.
- Behaviour Analysis / Behavioral Analysis: The study of behavioural patterns in data to derive actionable insights.
- Analytics: The systematic computational analysis of data.
- Customer Behaviour Analysis: The process of examining customer interactions to understand patterns and preferences.
- User Behaviour Analysis: Similar to customer behaviour analysis but focused on broader user interactions.
- Predictive Analytics: Techniques that use statistical algorithms and machine learning to predict future outcomes based on historical data.
- Real-Time Analytics: The process of analysing data as it is created or received.
- Log Analysis: The examination of log data to identify patterns or anomalies.
AI Search Behaviour Analysis Agents Redefining Analytics
AI search behaviour analysis agents are redefining analytics by delivering profound insights into user behaviour. From meticulous data collection to advanced predictive analytics, these systems empower organisations to optimise digital strategies and personalise customer experiences.
By harnessing AI technology, businesses gain a nuanced understanding of user behaviour, leading to enhanced customer engagement and more strategic decision-making.
Integrating advanced machine learning techniques, comprehensive log analysis, and state-of-the-art predictive analytics enables organisations to transform raw data into strategic actionable insights. This robust approach not only elevates customer satisfaction but also underpins sustainable innovation and long-term operational efficiency.
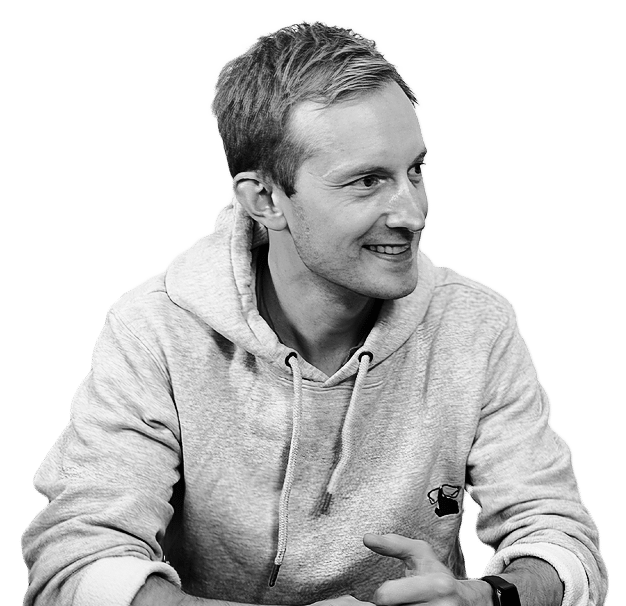